Artificial Intelligence (AI) agent software has become a crucial element for modern businesses, transforming how they operate, engage with customers, and automate essential processes. By streamlining routine tasks, enhancing customer support, and facilitating complex decision-making, AI agents have proven to be invaluable assets for organizations aiming to improve productivity and customer experience.
As demand for AI-driven solutions rises, businesses are increasingly integrating these intelligent systems into their daily operations. Companies in industries such as healthcare, retail, finance, and logistics have all embraced AI agents to achieve higher efficiency and drive customer satisfaction.
Organizations are leveraging AI agents to provide 24/7 customer support, enabling businesses to meet customer expectations for timely and accurate responses. By automating customer interactions, businesses can reduce response times, handle higher query volumes, and free up human agents to focus on more complex tasks.
Additionally, AI agents play a vital role in data analysis, allowing businesses to gain actionable insights from large datasets, predict customer behaviors, and improve decision-making processes.
Key Takeaways
- AI agent software is instrumental in automating business processes and enhancing customer support.
- High-quality, accurate data is essential for the effectiveness of AI agents.
- Smooth integration with existing systems is vital to ensure seamless workflows.
- Organizations must plan for scalability and performance as demand for AI agents increases.
- User adoption is supported by training and proactive change management strategies.
- Advances in Natural Language Processing (NLP) are improving AI’s ability to handle language nuances and multilingual support.
- Ethical considerations and regulatory compliance are becoming a key focus in AI development.
- Cybersecurity measures such as encryption and access controls help safeguard AI systems from threats.
- A strategic approach involving training, performance monitoring, and constant improvement is essential for successful AI implementation.
Understanding the Role of AI Agent Software
AI agent software refers to intelligent programs that can learn, reason, and make decisions. These agents are designed to assist users in tasks ranging from customer support to data analysis. Businesses in industries such as healthcare, retail, and finance are leveraging AI agents to automate operations, improve service delivery, and reduce costs. The role of AI agents is constantly expanding.
Companies are using these systems to provide 24/7 support, predict customer needs, and drive personalized user experiences. As AI capabilities grow, the potential applications of AI agents will continue to expand.
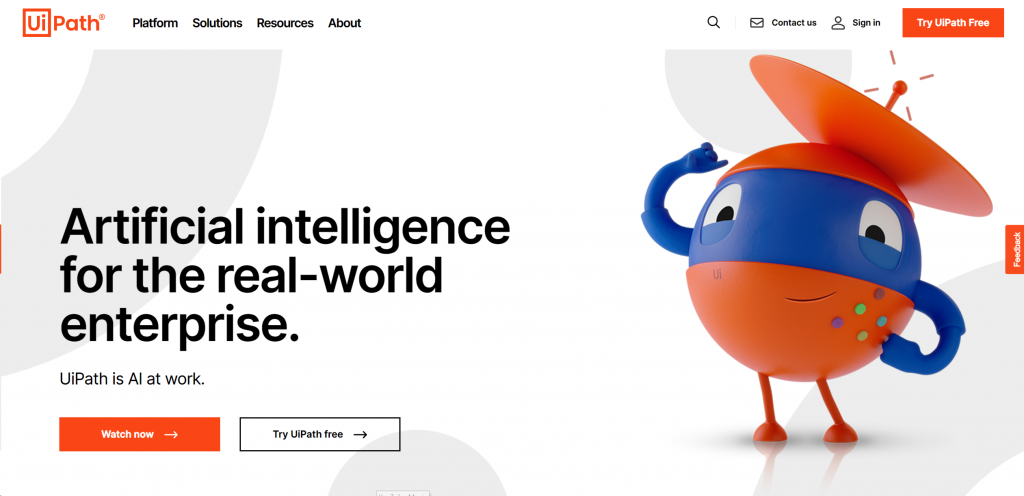
Common Challenges in AI Agent Software
Data Quality and Availability
Challenge
AI agents rely on large datasets to learn and make accurate predictions. If the data is incomplete, biased, or outdated, the agent’s performance can be affected.
Impact
The impact of poor data quality extends to decision-making errors, suboptimal customer support, and compliance issues. Poor-quality data can also distort AI predictions, affecting business performance and customer satisfaction.
Solution
To address data quality issues, organizations should:
- Implement data management protocols to ensure data accuracy and completeness.
- Use automated data cleansing tools to remove duplicates and inconsistencies.
- Conduct regular audits of data sources to maintain data integrity.
- Prioritize access to fresh, relevant, and diverse datasets to improve the AI’s decision-making capabilities.
Integration with Existing Systems
Challenge
Integrating AI agents with current systems, platforms, and databases is often a complex process. Disparate systems may use different data formats or protocols, causing compatibility issues.
Impact
Integration challenges can result in increased development time, operational delays, and disruptions to existing workflows. System silos may form, reducing the effectiveness of the AI agent.
Solution
To ensure smooth integration, companies should:
- Conduct a system compatibility assessment before selecting an AI agent solution.
- Utilize APIs and middleware to establish communication between systems.
- Choose AI agent software with pre-built integration capabilities.
- Involve IT teams and software developers early in the integration process to resolve issues as they arise.
Scalability and Performance
Challenge
As businesses grow, AI agents must handle an increasing number of interactions and users. This growth can place a strain on infrastructure and system resources.
Impact
Poor scalability can lead to system slowdowns, service interruptions, and higher operational costs. If AI agents fail to manage large volumes of interactions, customer experience may be negatively affected.
Solution
To achieve scalability, businesses can:
- Use cloud-based AI agent platforms that offer dynamic resource allocation.
- Implement load balancing mechanisms to distribute workloads efficiently.
- Conduct performance stress tests to identify and resolve potential bottlenecks.
- Design the system with scalability in mind from the outset.
User Adoption and Change Management
Challenge
The introduction of AI agents may raise concerns among employees, particularly regarding job security and the complexity of learning new technology.
Impact
Low employee adoption rates may reduce the overall effectiveness of AI agent software. Without proper usage, businesses may face delays in achieving their expected return on investment.
Solution
To drive user adoption, companies can
- Offer comprehensive training programs and workshops to familiarize employees with AI systems.
- Highlight case studies that demonstrate the benefits of AI in enhancing roles and simplifying tasks.
- Create simple, user-friendly interfaces for AI agent systems to make them accessible to non-technical users.
- Address employee concerns openly and involve them in the implementation process.
Ethical and Compliance Considerations
Challenge
AI agents are subject to ethical standards and regulatory compliance frameworks that aim to ensure fairness, transparency, and data privacy.
Impact
Failure to meet compliance requirements may expose businesses to legal risks and reputational damage. Ethical issues, such as AI bias, may reduce public trust in the technology.
Solution
To ensure ethical AI practices, organizations should:
- Develop explainable AI models that provide clear reasoning for AI-driven decisions.
- Mitigate bias in AI training data by using diverse, representative datasets.
- Establish AI governance frameworks that prioritize fairness, accountability, and transparency.
- Stay informed of new regulatory changes and ensure compliance with data protection laws.
Future Outlook – Enhancing AI Agent Software
Artificial Intelligence (AI) agent software is evolving rapidly, reshaping how businesses operate and interact with customers. From advancements in Natural Language Processing (NLP) to more robust ethical frameworks, the future of AI agents holds immense potential.
As businesses continue to integrate these intelligent tools into their operations, they must keep pace with emerging trends, explore future possibilities, and focus on key areas that will keep them ahead in this transformative space.
Advanced Natural Language Processing (NLP) Capabilities
Natural Language Processing (NLP) like IBM Watson is becoming more sophisticated, allowing AI agents to understand and respond to human language with greater precision. By recognizing slang, industry-specific jargon, and supporting multiple languages, AI agents are transforming user interactions.
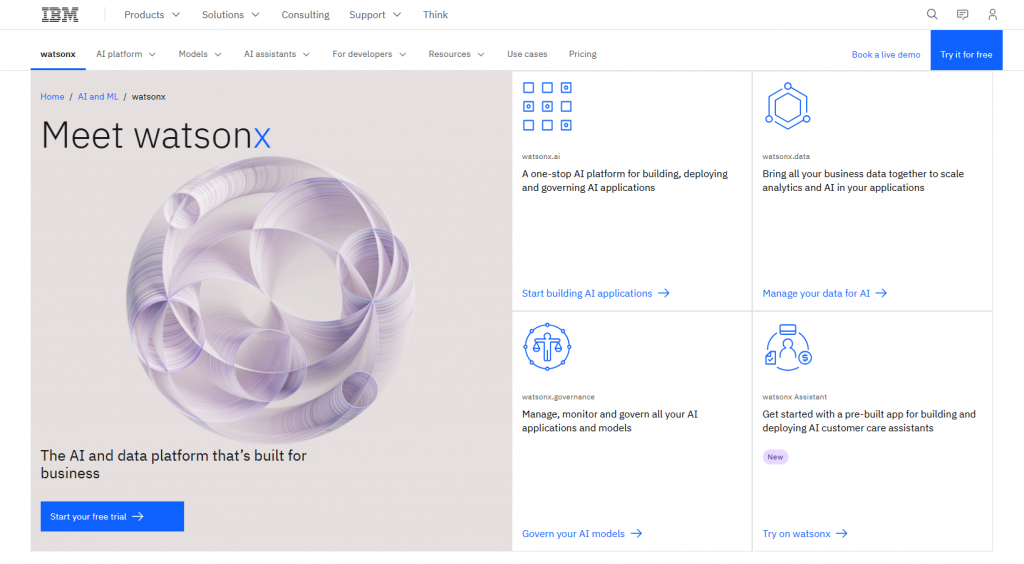
Next-generation NLP models, like OpenAI’s GPT models, are leading the charge in conversational AI. The future of NLP will see AI agents understanding the context of ongoing conversations more accurately, allowing for seamless, human-like interactions. Sentiment analysis will also play a pivotal role, enabling agents to detect emotions in user messages and provide empathetic responses.
Furthermore, AI agents will support a broader range of languages, empowering businesses to offer global customer support. Companies that prioritize NLP advancements will gain a competitive edge in customer experience and service delivery.
Hyperautomation with AI Agents
Hyperautomation involves automating as many processes as possible using AI and machine learning (ML). AI agents play a pivotal role in this movement by supporting end-to-end process automation. With hyperautomation, AI agents can connect workflows across multiple platforms, reducing the need for manual intervention.
This enables businesses to streamline complex operations, reduce operational costs, and improve overall efficiency. AI agents will also identify inefficiencies in workflows and suggest optimizations, which can lead to significant process improvements.
As part of this shift, AI agents will automatically perform repetitive tasks, such as scheduling appointments and sending reminders, freeing up human employees to focus on higher-value tasks. Hyperautomation is driving a future where business processes are faster, smarter, and more efficient.
Ethical AI and Explainability
As AI adoption grows, the focus on ethics and explainability has intensified. Users and regulatory bodies demand transparency in how AI agents make decisions. Businesses will prioritize explainable AI (XAI) where AI models can clearly explain their decision-making processes, which helps increase user trust and support regulatory compliance.
In addition, efforts to mitigate bias in AI models will become more pronounced. Developers will create more inclusive AI models that are trained on diverse datasets to reduce bias and promote fairness. AI governance frameworks will also gain importance as organizations seek to ensure responsible AI use.
These governance models will set clear standards for how AI is developed, deployed, and monitored. By fostering trust, reducing bias, and ensuring ethical AI practices, companies will be better positioned to meet regulatory expectations and earn customer trust.
AI-Driven Personalization
Personalization is becoming a key differentiator in customer experience. AI agents are increasingly leveraging vast amounts of customer data to deliver highly personalized experiences. This personalization extends to product recommendations, content suggestions, and tailored user interactions.
Hyper-personalized recommendations allow AI agent softwares to provide suggestions that align with user preferences, driving customer engagement and satisfaction. AI agents will also be able to map the customer journey, predicting customer needs at various touchpoints and offering proactive support.
Personalization will become more dynamic as agents learn from user behavior in real time, enabling companies to deliver experiences that are timely, relevant, and context aware. Businesses that prioritize AI-driven personalization will see stronger customer relationships and higher retention rates.
Edge AI for Real-Time Responses
With the rise of edge computing, AI agents can process data locally rather than relying solely on cloud-based processing. This shift accelerates response times and enhances user experiences. Edge AI enables on-device AI processing, meaning AI agents embedded in mobile apps or IoT devices can operate even without internet access.
This capability provides businesses with greater flexibility, especially in remote areas or environments with limited connectivity. By processing data locally, companies can also enhance data privacy since sensitive information never leaves the device.
Real-time responses are another key benefit, as local processing reduces latency, allowing AI agents to respond instantaneously. Businesses that embrace edge AI will benefit from faster response times, stronger data privacy, and the ability to deliver seamless interactions in offline scenarios.
No-Code and Low-Code AI Platforms
No-code and low-code platforms are democratizing AI development, allowing non-technical users to create and deploy AI agents. These platforms reduce the technical barrier to AI implementation, enabling business users, often referred to as citizen developers, to create custom AI workflows.
Companies that adopt these platforms will experience faster deployment times, as the process of building AI agents no longer requires extensive coding expertise. Low-code AI solutions also support customizable workflows, allowing businesses to design workflows that align with their specific operational needs.
This democratization of AI development accelerates the adoption of AI agents, empowers employees to be more involved in digital transformation, and drives faster time-to-market for AI-driven solutions.
Enhancing Customer Support with Conversational AI
Conversational AI agents are transforming customer support. These AI-driven assistants handle inquiries, resolve issues, and provide personalized assistance at scale. One of the biggest opportunities for businesses is offering 24/7 customer support, ensuring uninterrupted service for customers worldwide.
Proactive assistance is another key benefit, as predictive AI enables agents to identify potential customer needs before users even request help. Cost savings are also achieved through automated interactions, reducing the reliance on human support agents for routine queries.
Conversational AI can handle high volumes of inquiries simultaneously, leading to improved customer satisfaction and quicker issue resolution. Companies that leverage conversational AI in their customer support strategy will improve operational efficiency and elevate the customer experience.
AI Agents in Sales and Lead Generation
AI agents are driving growth in sales and lead generation by qualifying leads and nurturing prospects through automated workflows. Businesses are using AI-powered lead scoring to analyze lead behavior, prioritize follow-ups, and identify the most promising prospects.
By focusing on high-quality leads, companies can increase conversion rates and improve the efficiency of their sales teams. AI agents also streamline sales workflows, handling routine tasks such as follow-up emails, meeting scheduling, and reminders.
Predictive analytics allows AI agents to forecast customer purchasing behavior, enabling companies to tailor their sales strategies accordingly. Companies that leverage AI-driven sales strategies will experience increased lead generation, improved prospect engagement, and higher sales conversion rates.
AI Agents for Internal Process Automation
AI agents are reshaping internal processes by handling repetitive administrative tasks, improving efficiency, and reducing employee workload. In human resources (HR), AI agents assist with onboarding new hires, guiding them through the process and answering frequently asked questions.
This automated support enables HR teams to focus on strategic initiatives rather than administrative tasks. In IT support, AI agents provide instant assistance to employees, helping them resolve technical issues quickly and reducing the burden on IT helpdesk teams. Financial processes, such as invoice processing and approval workflows, are also being automated by AI agents.
Businesses that implement AI-driven internal process automation will experience faster task completion, cost savings, and a more engaged workforce. By freeing employees from repetitive tasks, companies can redirect human resources to higher-value projects that contribute to business growth.
Conclusion
While AI agent software presents immense opportunities for automation and efficiency, it also brings forth unique challenges. Issues like data privacy, system integration, scalability, and maintaining human oversight can impact the effectiveness of these tools.
To overcome these hurdles, businesses should prioritize data security protocols, adopt flexible software with API support, and ensure continuous training for employees and AI models. Addressing bias through diverse datasets and regular model audits is equally crucial to avoid skewed outcomes.
The key to success lies in a proactive approach—selecting the right AI agent software that aligns with business needs, investing in proper implementation, and regularly optimizing it for performance. Companies that address these challenges head-on can unlock the full potential of AI agents, driving greater productivity, improved customer experiences, and more informed decision-making.
What is AI agent software?
AI agent software refers to intelligent systems that automate tasks, support customer interactions, and assist in decision-making processes. These agents use machine learning and natural language processing to understand user inputs and deliver appropriate responses.
What are the key benefits of using AI agent software?
AI agent software improves efficiency, enhances customer service, enables 24/7 support, and reduces operational costs. It also supports process automation, boosts lead generation, and enhances personalization in customer interactions.
How do AI agents handle customer interactions?
I agents use conversational AI and natural language processing (NLP) to understand and respond to customer queries. They recognize user intent, offer solutions, and provide personalized responses based on user behavior and historical data.
What are the common challenges in implementing AI agent software?
Businesses face challenges such as data quality issues, system integration, user adoption, compliance, and scalability. Overcoming these challenges requires careful planning, advanced tools, and continuous system monitoring.