AI agents are becoming more popular, but what do they really do? Major tech companies like Google and Microsoft promote their AI agent products, but it can be confusing to understand what they mean.
AI agents are advanced software programs powered by artificial intelligence. They can analyze instructions, make decisions, and execute tasks independently. This sounds impressive, but how do AI agents actually work? This article will break down what AI agents are, how they function, and why they matter.
Key Takeaways
- AI agents are advanced software programs that can analyze instructions, make decisions, and execute tasks independently.
- AI agents can enhance productivity by automating tasks, reducing operational costs, and improving business processes, with benefits including up to 30% higher conversion rates.
- AI agents are used in various sectors, including marketing, commerce, and customer support, to provide personalized experiences, manage inventory, and offer multilingual support, with experts like Dr. Rachel Kim emphasizing their potential to transform industries and improve lives.
Defining AI Agents
AI agents are advanced artificial intelligence systems that can perform tasks autonomously without human intervention – making decisions based on data analysis and machine learning algorithms.
These intelligent agents can interact with humans through natural language processing – allowing them handle customer inquiries efficiently like human customer support agents would do traditionally without getting tired since machines do not get fatigued like humans who require rest after working hours are over each day across America!
AI Agents vs Non-Agentic Chatbots
Feature | AI Agents | Non-Agentic Chatbots |
---|---|---|
Core Functionality | Dynamic problem-solving capabilities driven by advanced algorithms. | Operates on predefined rules and scripts for basic tasks. |
Learning Capability | Can adapt and improve through interaction and machine learning. | Lacks learning ability; responses remain static. |
Autonomy | Functions independently, taking actions based on context. | No autonomy; relies strictly on pre-programmed responses. |
Complexity of Tasks | Handles multifaceted tasks like decision-making and advanced analytics. | Limited to simple tasks like answering FAQs or routing queries. |
Adaptability | Adjusts dynamically to new inputs or environments. | Static; cannot adjust to new scenarios or contexts. |
Technology Example | Powered by compound AI systems like Odin AI or IBM Watson Assistant. | Examples include basic FAQ bots or rule-based customer service bots. |
Real-World Case Study | Tesla’s Autonomous Driving AI learns and adapts to driving conditions. | E-commerce FAQ Bot answers common questions but cannot learn over time. |
How AI Agents Function
To understand how AI agents function, we can break down their operations into key stages:
Step 1: Identify the Use Case for AI Agents in Your Business
Before implementing AI agents, determine the specific challenges they will address within your business operations. AI agents can be used to automate repetitive tasks, enhance customer support, improve data processing, optimize decision-making, and personalize user experiences. Start by analyzing internal workflows to identify bottlenecks where automation can save time and resources.
Assess whether your business needs conversational AI, predictive analytics, process automation, or real-time decision-making capabilities. Engaging with different departments—such as sales, customer support, IT, and operations—can provide insights into where AI-driven solutions can create the most impact. A well-defined use case ensures that the AI agent aligns with business goals and delivers measurable value.
Step 2: Choose the Right AI Agent Framework or Platform
Selecting the right AI framework or platform is essential for building a reliable and scalable AI agent. The choice depends on factors like the complexity of the task, real-time processing needs, industry requirements, and budget. Businesses looking to develop conversational AI agents can opt for OpenAI’s GPT models, Google’s Dialogflow, or IBM Watson.
Companies needing workflow automation may prefer UiPath or Microsoft Azure AI, while those focused on data analytics and predictions can leverage TensorFlow, PyTorch, or Google Cloud AI. Other considerations include API availability, ease of integration with existing business tools, cloud compatibility, security, and compliance. Conducting pilot tests on different platforms helps ensure that the chosen AI solution meets performance and scalability requirements.
Step 3: Train the AI Model with Relevant Data
Once the AI agent framework is selected, the next step is training the model with high-quality data to improve accuracy and effectiveness. Data collection is crucial, as AI models learn from historical patterns and real-world inputs. Businesses must gather structured and unstructured data, including customer interactions, transaction records, chatbot conversations, or workflow logs. If using supervised learning, the data must be labeled and categorized so that the AI can recognize patterns accurately.
Unsupervised learning methods can also be used when dealing with large datasets that require anomaly detection or clustering. Training is an iterative process—AI agents must be tested, fine-tuned, and retrained regularly to ensure optimal performance. Reinforcement learning techniques, where the AI improves through trial and error, can further enhance decision-making capabilities.
Step 4: Deploy AI Agents in Real-World Applications
After training, the AI agent must be deployed into the appropriate business environment. Deployment can take various forms, such as chatbots on customer support platforms, virtual assistants in enterprise software, predictive models in analytics dashboards, or automation tools for backend operations. A phased rollout approach is often recommended—starting with a controlled test environment where initial user interactions can be monitored for improvements.
Integration with existing systems (such as CRMs, ERP solutions, or cloud services) is essential for seamless operation. Businesses should ensure that deployment includes robust security protocols to protect data privacy and maintain compliance with regulations such as GDPR and HIPAA. Real-time monitoring of AI interactions helps identify issues and allows for immediate adjustments to enhance user experience.
Step 5: Monitor and Improve AI Agent Performance
The work doesn’t end after deployment; ongoing monitoring and optimization are critical for long-term AI success. Businesses should track key performance indicators (KPIs) such as response accuracy, processing speed, customer satisfaction scores, conversion rates, and task completion efficiency. Advanced analytics dashboards and A/B testing help assess AI performance in different scenarios. Gathering user feedback is equally important—customers and employees interacting with the AI agent should have an easy way to report inconsistencies, errors, or improvements.
Periodic retraining with new data ensures that the AI model stays updated with evolving trends and business needs. Companies can also implement human-in-the-loop (HITL) approaches, where human oversight is used to refine AI decision-making in complex cases. Continuous enhancements help AI agents remain efficient, reduce errors, and improve their ability to provide valuable insights and automation.
Final Thoughts
Successfully implementing AI agents requires a strategic approach that includes defining the right use case, selecting the best platform, training models with high-quality data, deploying seamlessly into business operations, and continuously optimizing performance. AI agents are revolutionizing industries by automating tasks, improving customer interactions, and enhancing decision-making processes. Businesses that leverage AI-driven solutions effectively will gain a competitive edge, drive operational efficiency, and provide superior user experiences.
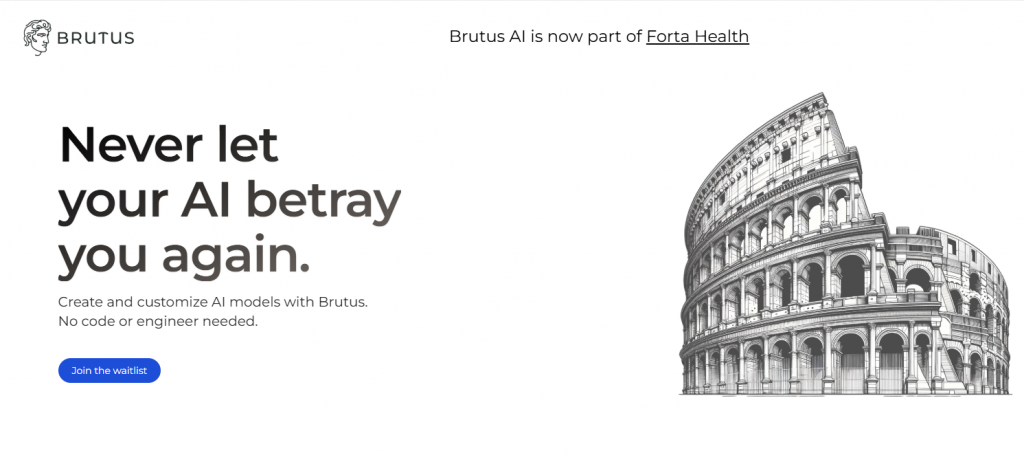
The Synergy of These Stages
The true power of AI agents lies in the synergy of these stages. From gathering data to executing tasks, each step builds upon the previous one to create a cohesive system capable of solving complex problems.
Whether it’s Tesla’s Autopilot navigating busy streets or ChatGPT engaging users in meaningful conversations, the seamless integration of perception, interpretation, decision-making, action, learning, and integration ensures that AI agents remain indispensable tools in modern society.
The Role of AI Agents in Different Sectors
AI agents are playing transformative roles across various industries, solving complex challenges, improving efficiency, and delivering personalized experiences. By automating tasks, analyzing data, and making intelligent decisions, AI agents have become indispensable in numerous sectors. Here’s an in-depth look at their roles in four key industries:
Healthcare – Revolutionizing Diagnostics and Patient Care
Challenges in Healthcare
Healthcare providers face issues such as delayed diagnosis, administrative inefficiencies, and a growing demand for personalized patient care. Traditional methods often rely on manual analysis, leading to errors and time delays.
Role of AI Agents
AI agents are deployed to address these issues effectively:
• Diagnostic Accuracy: AI agents like IBM Watson Health analyze medical images, detect anomalies, and assist in early disease diagnosis.
• Patient Engagement: Virtual health assistants provide 24/7 support, answering patient queries and scheduling appointments.
• Operational Efficiency: AI tools streamline administrative processes such as billing, coding, and patient record management.
Case Study – Mount Sinai Hospital
• The Problem: Mount Sinai Hospital struggled with early detection of diseases like liver cancer.
• The Solution: The hospital adopted AI tools that used machine learning to scan electronic health records (EHR) and detect subtle patterns.
• The Outcome: The system achieved 94% accuracy in identifying high-risk patients, reducing hospital readmissions by 15%. This not only improved patient outcomes but also cut operational costs.
Explore more about Mount Sinai’s AI-driven healthcare solutions.
E-Commerce – Enhancing Customer Experience and Operations
Challenges in E-Commerce
Online retailers often face challenges such as high return rates, inventory mismanagement, and the need to deliver personalized shopping experiences to retain customers.
Role of AI Agents:
AI agents have become integral to e-commerce platforms by:
• Personalized Recommendations: They analyze customer behavior and purchase history to suggest relevant products.
• Inventory Optimization: AI tools predict demand trends and optimize stock levels to reduce overstocking or shortages.
• Fraud Prevention: Advanced algorithms detect and flag suspicious transactions, ensuring secure shopping experiences.
Case Study: ASOS
• The Problem: ASOS experienced high return rates and inconsistent customer retention.
• The Solution: The company implemented AI-driven recommendation engines and virtual try-on tools to provide tailored product suggestions.
• The Outcome: ASOS reduced return rates by 20% and saw a 25% increase in repeat purchases, boosting overall sales revenue by 15%.
Learn more about ASOS’s AI-powered strategies.
Finance: Strengthening Fraud Detection and Customer Interaction
Challenges in Finance
Financial institutions face increasing threats from fraud, compliance requirements, and the need for personalized customer experiences.
Role of AI Agents
AI agents address these challenges with precision:
• Fraud Detection: AI systems like Mastercard’s Decision Intelligence monitor transaction patterns to detect anomalies and prevent fraudulent activities.
• Customer Support: Chatbots offer instant assistance with account-related queries, improving customer satisfaction.
• Risk Assessment: AI tools analyze vast datasets to assess creditworthiness and market risks, ensuring informed decision-making.
Case Study: HSBC
• The Problem: HSBC struggled with rising fraud cases and regulatory compliance.
• The Solution: They implemented an AI agent with anomaly detection capabilities to monitor transactions in real time and identify potential risks.
• The Outcome: HSBC reduced fraudulent activities by 30% and achieved a 95% accuracy rate in anomaly detection, ensuring stronger compliance and customer trust.
Discover how HSBC leverages AI for fraud detection.
Marketing – Optimizing Campaigns and Audience Engagement
Challenges in Marketing
Marketing teams often grapple with challenges like targeting the right audience, measuring campaign success, and delivering personalized experiences at scale.
Role of AI Agents
AI agents are redefining marketing strategies through:
• Audience Segmentation: AI tools analyze customer data to create highly targeted audience segments.
• Campaign Automation: Agents manage multichannel campaigns, delivering the right message at the right time.
• Predictive Analytics: AI predicts customer behavior, allowing marketers to fine-tune strategies for maximum impact.
Case Study: Coca-Cola
• The Problem: Coca-Cola aimed to optimize its digital marketing campaigns for global audiences.
• The Solution: The company used AI-powered tools to analyze consumer data and identify trends. These insights were used to create personalized campaigns.
• The Outcome: Campaign engagement rates increased by 35%, and the company strengthened its brand presence in emerging markets.
Learn more about AI in Coca-Cola’s marketing.
Challenges with AI Agents
Data Privacy and Security Concerns
AI agents process vast amounts of sensitive data, making data privacy and security critical concerns. Mismanagement can lead to breaches, regulatory penalties, and loss of trust.
Key Issues
• Sensitive Data Handling: AI agents in sectors like healthcare and finance process personal information, increasing vulnerability to cyberattacks.
• Regulatory Compliance: Laws like GDPR and CCPA require stringent data handling practices, posing a challenge for organizations.
Case Study: Healthcare and Data Privacy
A healthcare provider implemented AI agents to streamline patient record management. Despite improved efficiency, they faced initial challenges in ensuring data encryption and HIPAA compliance. By adopting advanced encryption tools and AI monitoring systems, the provider safeguarded patient data, reinforcing trust and regulatory adherence.
Implementation Complexity
Deploying AI agents often involves integrating advanced systems into existing infrastructure, which can be technically demanding.
Key Issues
• Integration with Legacy Systems: Many organizations operate on older systems, making AI integration complex.
• Skill Gap: Employees may lack the technical expertise to operate or manage AI agents effectively.
Case Study: Banking Industry Transformation
A global bank implemented AI agents for fraud detection. The challenge was integrating the system into their legacy infrastructure. They overcame this by partnering with a tech consultancy, which designed custom APIs to bridge old systems with the new AI platform, reducing fraud by 35% within a year.
Dependence on Quality Training Data
AI agents rely on high-quality datasets for training. Inadequate or biased data can lead to inaccurate outputs, affecting decisions and outcomes.
Key Issues
• Data Quality: Poor datasets lead to unreliable predictions or biased recommendations.
• Bias in Training Data: Unbalanced datasets can propagate systemic biases, impacting sectors like recruitment or lending.
Case Study: Recruitment Bias Challenge
A recruitment company implemented an AI agent to screen resumes. Initial outcomes showed a bias favoring certain demographics due to an unbalanced training dataset. By retraining the system with diverse, anonymized datasets, the company improved hiring fairness and increased applicant satisfaction by 40%.
Ethical Considerations and Transparency
As AI agents make autonomous decisions, ensuring ethical behavior and transparency becomes paramount.
Key Issues:
• Decision-Making Bias: Unclear decision-making processes can lead to ethical dilemmas.
• Accountability: Determining responsibility for AI errors can be challenging.
Case Study: AI in Law Enforcement
NYPD used AI agents for crime prediction. Concerns arose regarding potential racial bias in the system’s recommendations. By implementing regular audits and incorporating community feedback, they ensured more ethical and balanced outputs.
Opportunities with AI Agents
Enhanced Efficiency and Productivity
AI agents automate repetitive tasks, enabling faster and more accurate operations.
Key Benefits
• Streamlined Operations: Automating routine tasks reduces human error and saves time.
• Cost Savings: Efficient resource allocation leads to lower operational costs.
Case Study: Retail Inventory Management
A leading retailer adopted AI agents for inventory tracking. The system reduced stockouts by 25% and cut inventory holding costs by 15% by predicting demand patterns with high accuracy.
Improved Decision-Making
AI agents process large datasets in real time, providing actionable insights for informed decision-making.
Key Benefits
• Data-Driven Strategies: AI-powered insights optimize marketing, sales, and logistics.
• Predictive Analytics: AI forecasts trends, helping businesses adapt proactively.
Case Study: AI in Agriculture
A farming cooperative utilized AI agents for precision agriculture. By analyzing weather, soil, and crop data, the system optimized irrigation and fertilizer use, boosting yields by 20% while conserving resources.
Scalable Growth
AI agents support business scalability by automating processes and adapting to increased demands.
Key Benefits
• Flexibility: AI systems scale effortlessly to meet growing organizational needs.
• Global Reach: Multilingual AI agents enable expansion into diverse markets.
Case Study: E-Commerce Expansion
An e-commerce platform used AI chatbots for global customer support. By offering services in multiple languages and handling high query volumes, they scaled operations across five new markets, increasing revenue by 30% in a year.
Innovative Customer Experiences
AI agents enable personalized interactions, enhancing customer satisfaction and loyalty.
Key Benefits
• Personalization: Tailored recommendations improve user experience.
• 24/7 Availability: AI-powered systems ensure round-the-clock support.
Case Study: Hospitality Industry
A luxury hotel chain integrated AI agents for concierge services. The system personalized recommendations for dining and activities, based on guest preferences. Customer satisfaction scores rose by 20%, leading to increased repeat bookings.
Conclusion
AI agents are transforming industries by automating tasks, improving decision-making, and driving scalable growth. From retail and healthcare to finance and marketing, these advanced systems are addressing complex challenges and creating opportunities for innovation. While hurdles like data privacy and implementation complexities exist, the advantages—such as enhanced efficiency, productivity, and personalized experiences—are undeniable.
By carefully integrating AI agents into workflows and leveraging their capabilities, businesses can unlock significant potential for growth and improved customer satisfaction. As technology evolves, AI agents will play an even more critical role in shaping a connected and intelligent future, redefining how industries operate and creating lasting value.
Frequently Asked Questions
What are AI agents – and why are they important?
AI agents are computer programs, they help people, and make things easier… like a tool, they can do many tasks, and they matter because they can think, and learn.
How do AI agents work – and what can they do?
AI agents work with data, and use it to make decisions, they can talk to people, answer questions, and even solve problems… like a person, but faster, and more accurate.
Can AI agents think – and learn like humans?
AI agents can think and learn, but not like humans… they use special codes and math to make decisions and get better at what they do, and they can even help people make good choices.
Why are AI agents useful – and how can we use them?
AI agents are useful, because they can help people, with many things… like answering questions, solving problems, and even doing tasks, that are hard, or boring, and we can use them, to make our lives easier, and more fun.